AI and CRISPR – A New Frontier in Precision Biology
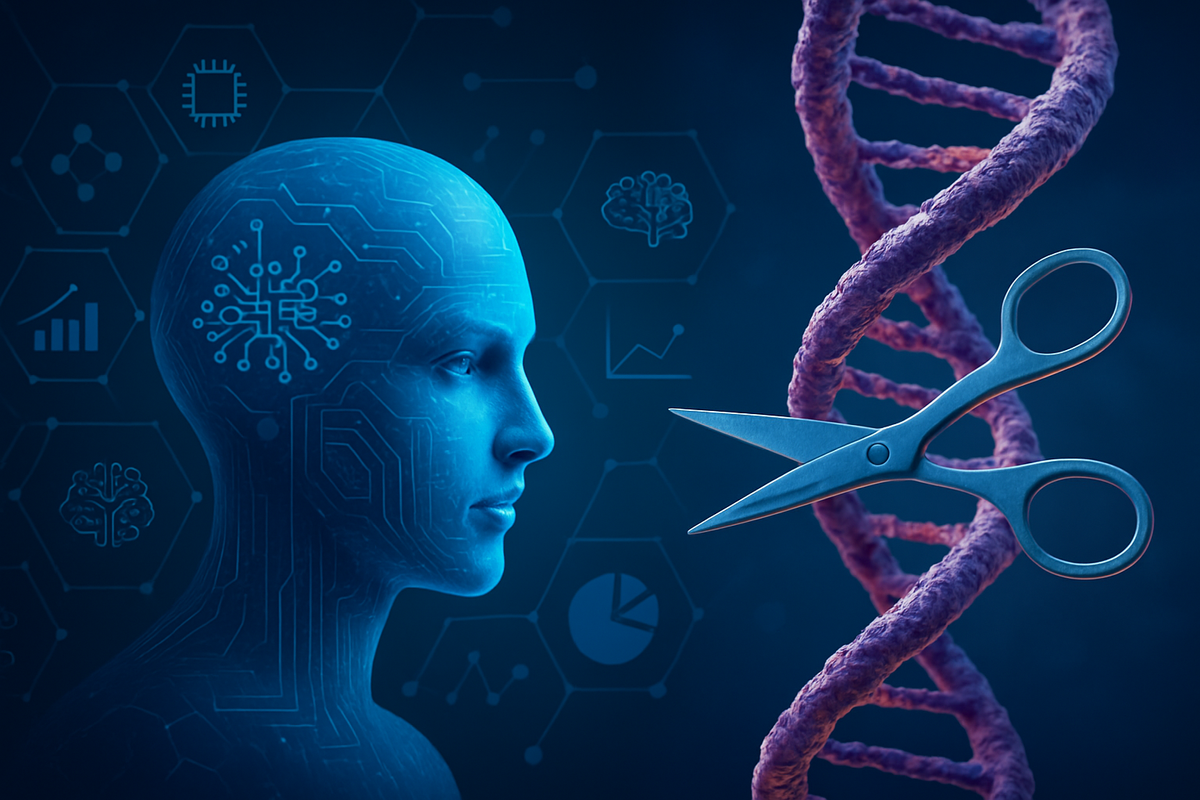
This post is also available as a podcast if you prefer to listen on the go or enjoy an audio format:
For those unfamiliar, CRISPR (Clustered Regularly Interspaced Short Palindromic Repeats) is a natural genetic system found in bacteria that has been adapted as a powerful gene-editing technology. It allows scientists to precisely alter DNA by using a specialized protein (commonly Cas9) guided by a customizable RNA sequence to cut specific locations in a genome, enabling inserting, deleting, or modifying genetic material.
The marriage of artificial intelligence and CRISPR gene-editing technologies has resulted in a transformative era in biotechnology, offering a promising future. This union brings together computational power and biological precision in ways that were unimaginable just a decade ago. While each technology has independently revolutionized its field, AI in data processing and decision-making, CRISPR in genetic modification, their integration creates something greater than the sum of their parts. Together, they are reshaping our approach to human health, agriculture, and synthetic biology, offering solutions to challenges that once seemed insurmountable.
AI's exceptional abilities in pattern recognition, data analysis, and predictive modeling complement the intricate task of editing genomes with remarkable synergy. CRISPR technology has given scientists unprecedented precision in cutting and modifying specific DNA sequences. However, the complexity of genomic interactions means that selecting optimal editing targets, predicting unintended effects, and anticipating biological outcomes remain formidable challenges. This is where artificial intelligence transforms a powerful but imperfect tool into something approaching biological mastery.
The genomic landscape is vast and intricate, with billions of base pairs and countless potential interaction points. Machine learning models trained on extensive genomic datasets now identify ideal CRISPR targets with greater accuracy than human researchers alone could achieve. These AI systems continuously improve their predictions by analyzing outcomes from thousands of experiments, learning which edits succeed and which create unwanted effects. Deep learning algorithms simulate protein-DNA interactions and predict the consequences of genetic modifications before they occur in a laboratory setting, reducing the need for trial-and-error experimentation.
This AI enhancement addresses CRISPR's most significant limitations: off-target effects and efficiency, providing a sense of reassurance. By analyzing the entire genome, AI systems can identify sequences like the target that might be accidentally edited, allowing researchers to redesign their approach. The result is not just faster research but fundamentally safer genetic interventions. What once took months of painstaking laboratory work can now be accomplished in days or even hours, with higher confidence in the outcomes. This acceleration enables researchers to explore genetic solutions to problems at unprecedented speed, from disease treatments to crop improvements.
Perhaps nowhere is the impact of AI-enhanced CRISPR more evident than in personalized medicine. Traditional medical treatments often follow a one-size-fits-all approach, but genetic diseases manifest differently based on individual genomic variations. By analyzing patient-specific genetic data, AI algorithms can suggest personalized CRISPR edits tailored to an individual's unique genomic profile.
This approach has already shown promise for conditions previously considered untreatable. For rare genetic disorders like Duchenne muscular dystrophy, some forms of blindness, and sickle cell anemia, AI-guided CRISPR therapies are moving from theoretical possibilities to clinical trials. The algorithms identify where to edit and how to deliver the CRISPR system to target cells effectively. These personalized treatments represent a fundamental shift in medicine, from managing symptoms to addressing the genetic root causes of disease.
Beyond human health, the synergy between AI and CRISPR is transforming agriculture in ways that address some of humanity's most pressing challenges. Climate change, population growth, and resource constraints demand crops that can produce more food with fewer resources. AI-driven CRISPR applications enable plants to develop enhanced resilience to drought, heat, pests, and disease.
Unlike traditional genetic modification, which often involves introducing genes from different species, CRISPR typically makes precise changes to a plant's existing DNA. AI helps identify which specific genetic sequences to target to achieve desired traits while minimizing disruption to other plant functions. For example, researchers have used this approach to develop wheat varieties resistant to powdery mildew, rice that can withstand flooding, and tomatoes with enhanced nutritional profiles. These innovations promise to improve food security while reducing the environmental impact of agriculture through decreased pesticide use and improved resource efficiency.
The extraordinary power of AI-enhanced gene editing brings profound ethical, social, and governance questions that society must address. The ability to edit human embryos, modify entire ecosystems, or create synthetic organisms requires careful consideration of potential consequences, both intended and unintended. The quick pace of technological advancement often outstrips our ability to develop appropriate oversight mechanisms, creating regulatory gaps that could lead to misuse or harm.
AI's "black box" nature compounds these challenges. Many advanced machine learning models operate in ways that are not fully transparent, even to their creators. Understanding why specific changes were suggested becomes crucial when these systems recommend genetic edits. Without this transparency, we risk making permanent genetic alterations based on flawed or biased algorithms. Developing explainable AI for genetic applications must be a priority for researchers in both fields.
As these technologies become more refined and accessible, questions of equity and access move to the forefront. Who controls these powerful tools, and who benefits from them? The knowledge and resources required to implement AI-enhanced CRISPR systems remain concentrated in wealthy institutions and nations. Without deliberate efforts to broaden access, these technologies could exacerbate existing healthcare and agricultural disparities rather than alleviate them.
At the same time, democratization brings its challenges. As the technical barriers to gene editing continue to fall, governance frameworks must evolve to prevent misuse while enabling beneficial applications. Engaging diverse stakeholders, scientists, ethicists, policymakers, and the public requires ongoing dialogue about appropriate limits and safeguards. A select few should not make decisions about modifying the genetic code that shapes all life, but should reflect broader societal values and priorities.
The fusion of AI and CRISPR represents one of our most significant technological convergences. It offers unprecedented opportunities to address disease, hunger, and environmental challenges. Yet realizing this potential requires more than technical advancement; it demands wisdom in application and foresight in governance.
The path forward lies in responsible innovation: developing these technologies with built-in safeguards, transparent processes, and inclusive decision-making. This means investing in technical capabilities, ethical frameworks, regulatory systems, and public engagement. It also means fostering collaboration across disciplines and borders to ensure that benefits are widely shared and risks are carefully managed.
The future of precision biology will be shaped by how powerful our tools become and how thoughtfully we deploy them. By embracing both scientific ambition and ethical reflection, we can harness the transformative potential of AI-enhanced CRISPR to create a healthier, more sustainable world, one precise edit at a time.
BearNetAI remains committed to exploring these complex questions and bringing clarity to our community as the digital frontier evolves. By fostering nuanced conversation now, before verified agent technology becomes ubiquitous, we can guide its development in ways that enhance human capability while preserving authentic connection and individual autonomy.
The future of digital identity and AI representation isn't predetermined. It will be shaped by the choices we make, the standards we establish, and the values we prioritize as we navigate this remarkable technological transition. That future remains ours to create, provided we approach these questions with the thoughtfulness and foresight they deserve.
BearNetAI, LLC | © 2024, 2025 All Rights Reserved
Support BearNetAI
BearNetAI exists to make AI understandable and accessible. Aside from occasional book sales, I receive no other income from this work. I’ve chosen to keep BearNetAI ad-free so we can stay independent and focused on providing thoughtful, unbiased content.
Your support helps cover website costs, content creation, and outreach. If you can’t donate right now, that’s okay. Sharing this post with your network is just as helpful.
Thank you for being part of the BearNetAI community.
Books by the Author:
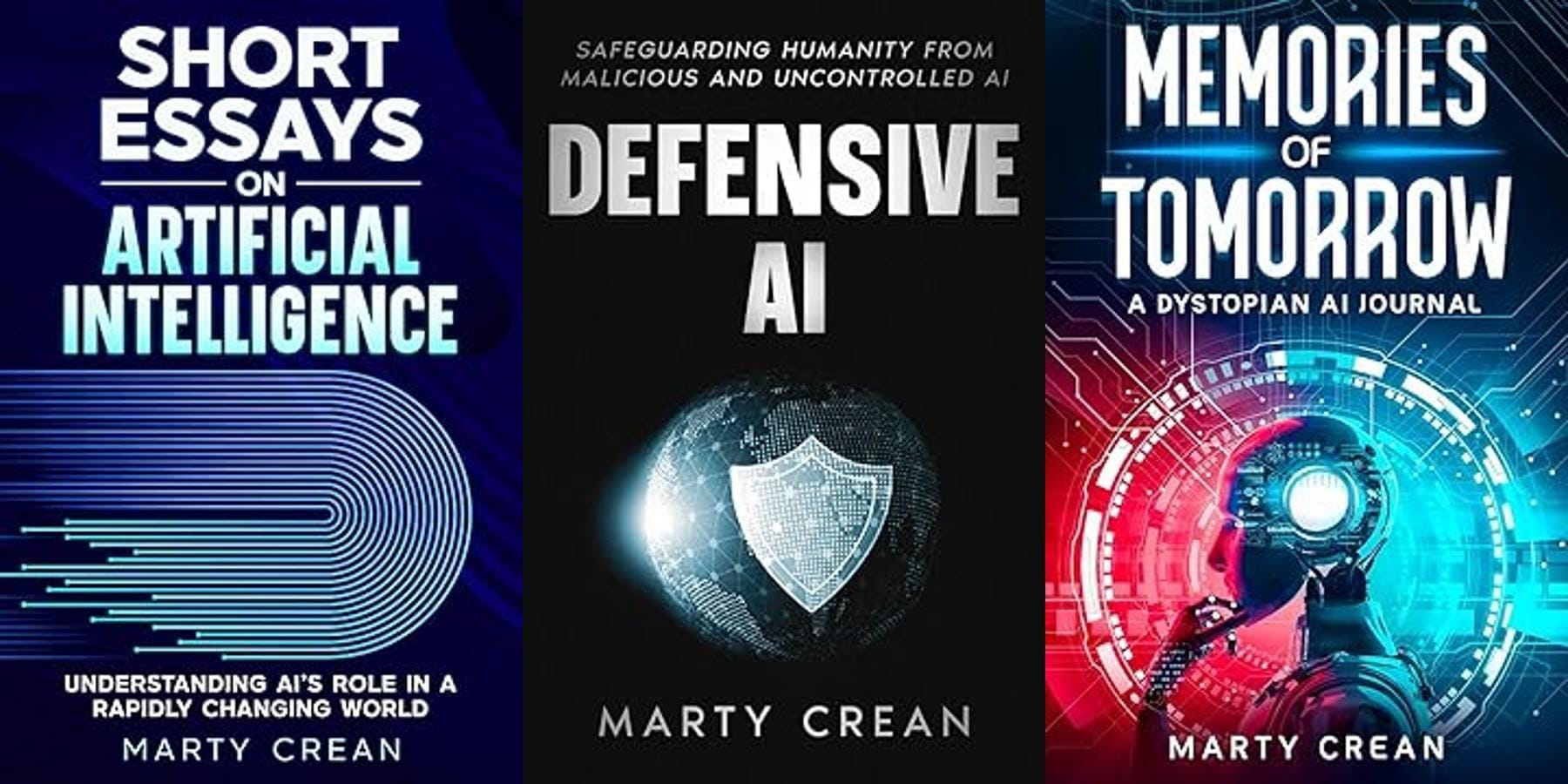
Categories: AI Ethics, Digital Identity, AI Governance, Human-AI Interaction, Technology and Society
Glossary of AI Terms Used in this Post
Algorithm: A set of rules or instructions a computer follows to solve a problem or perform a task.
Bias: Systematic and unfair discrimination in AI outcomes due to flawed data or algorithms.
Black Box: A system whose internal workings are not visible or understandable, making its decision-making process opaque.
Dataset: A structured data collection used for training or evaluating AI models.
Deep Learning: A subset of machine learning involving neural networks with many layers, used for complex tasks like image or speech recognition.
Feature Extraction: Transforming raw data into meaningful input variables for an AI model.
Generalization: The ability of an AI model to apply what it has learned from training data to new, unseen data.
Inference: Using a trained AI model to make predictions or decisions based on new input data.
Machine Learning: A branch of AI where systems learn patterns from data to make decisions without being explicitly programmed.
Model Training: The process of teaching an AI system to recognize patterns in data through iterative learning.
Neural Network: A model inspired by the human brain’s structure, consisting of layers of nodes (neurons) that process data.
Overfitting: A modeling error where an AI learns noise in the training data rather than the underlying pattern, reducing its accuracy on new data.
Predictive Modeling: Using statistical and machine learning methods to forecast outcomes based on input data.
Supervised Learning: A machine learning method that trains the model on labeled data.
Transparency: The degree to which the operations of an AI system can be understood and interpreted by humans.
Citations:
Anzalone, A. V., Koblan, L. W., & Liu, D. R. (2020). Genome editing with CRISPR–Cas nucleases, base editors, transposases, and prime editors. Nature Biotechnology.
Chen, C. Y., & Rostami, M. (2022). Machine Learning Applications in CRISPR Off-Target Prediction. Computational and Structural Biotechnology Journal.
Chung, J., et al. (2021). DeepCRISPR: Optimized CRISPR guide RNA design using deep learning. Nature Communications.
He, Y., & Wang, Y. (2021). AI Meets Genome Editing: Advances and Challenges. Trends in Biotechnology.
Hsu, P. D., Lander, E. S., & Zhang, F. (2014). Development and applications of CRISPR-Cas9 for genome engineering. Cell.
Kim, H. K., et al. (2020). Predicting the efficiency of CRISPR-Cas9 guide RNAs using a deep learning model. Nature Biotechnology.
Zhou, Y., et al. (2023). Ethical implications of AI-CRISPR convergence: A call for international governance. AI and Society.
LinkedIn BlueskySignal: bearnetai.28