AI and the “Running out of Data” Problem
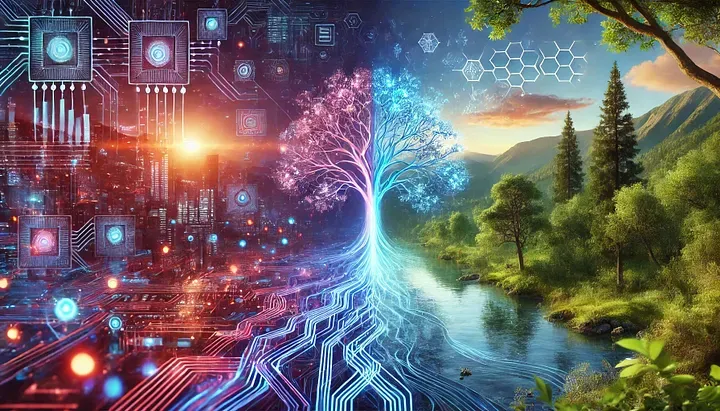
This week’s post is also available as a podcast if you prefer to listen on the go or enjoy an audio format:
Artificial Intelligence has become central to modern technological advancement, influencing everything from medical diagnostics to self-driving vehicles. The success of AI systems stems mainly from their ability to learn from massive datasets. However, a growing concern has emerged: AI systems may be approaching the limits of high-quality training data. Combined with the rise of synthetic data generation, this limitation presents opportunities and challenges for the field’s future.
The Internet contains vast quantities of information, but not all of it serves as valuable training material for AI systems. High-quality data — characterized by accuracy, diversity, and relevance — exists in limited quantities. Preparing data for AI training involves extensive cleaning and organization, which further reduces the pool of usable information. This constraint becomes increasingly apparent as AI models grow in size and complexity.
In specialized fields like healthcare, data scarcity poses challenges. Patient privacy regulations restrict data sharing while the need for accurate, comprehensive medical information grows. Even in areas with abundant data, AI systems often encounter diminishing returns — adding similar information yields progressively minor performance improvements.
Legal and ethical considerations add another layer of complexity. Questions about data ownership, consent, and privacy rights limit the collection and use of certain types of information. These constraints affect AI development across industries, from financial services to autonomous transportation.
Researchers and developers have turned to synthetic data — artificially generated information that mirrors real-world patterns to address these challenges. This approach offers several advantages, such as privacy protection and unlimited data generation, while introducing its own set of considerations, such as potential biases and limitations in mirroring complex real-world scenarios.
Synthetic data generation takes multiple forms. Computer simulations create virtual environments for testing autonomous systems. Advanced AI models produce realistic images, text, and audio that capture essential patterns from accurate data. Researchers also employ mathematical models to generate specialized datasets for specific applications, such as financial forecasting or medical research.
Synthetic data offers distinct benefits for AI development. It allows researchers to protect privacy by replacing sensitive information with artificial alternatives. Healthcare organizations can share synthetic patient records that maintain statistical relevance while preserving confidentiality.
The ability to generate unlimited amounts of data helps overcome scarcity issues. AI systems can train on larger datasets, potentially improving their performance and reliability. Developers can also create balanced datasets that help reduce bias in AI systems.
Another key advantage is creating data for rare events. Self-driving car systems can learn from simulated accidents without waiting for real-world incidents, and cybersecurity teams can generate examples of network attacks to improve threat detection systems.
However, synthetic data has limitations. Generated data may miss subtle real-world patterns, leading to AI systems that perform well in testing but struggle in actual applications. If the generation process relies on biased source data, the synthetic output may reinforce existing prejudices.
AI systems trained primarily on synthetic data risk becoming too specialized, potentially failing to handle real-world variations effectively. Questions about ownership and regulation of synthetic datasets remain unresolved, creating uncertainty for organizations investing in this technology.
The future of AI development likely requires effectively combining real and synthetic data. This mixed approach can maximize the benefits of both types while minimizing their drawbacks. Success depends on several key factors: improving the quality of both real and synthetic datasets, developing clear ethical guidelines for data generation and use, and advancing the technology that creates synthetic information.
Collaboration across disciplines is not just beneficial but essential. To navigate technical and societal challenges, computer scientists must work with domain experts, ethicists, and legal professionals. This cooperative effort, including your valuable contribution, will help shape responsible practices for developing and deploying AI systems in an environment of limited natural data.
The path forward involves not just creating, validating, and using data, but doing so with careful consideration. As AI continues influencing more aspects of daily life, the decisions we make about data become increasingly essential for society.
Thank you for being a part of this fascinating journey.
BearNetAI. From Bytes to Insights. AI Simplified.
BearNetAI is a proud member of the Association for the Advancement of Artificial Intelligence (AAAI), and a signatory to the Asilomar AI Principles, committed to the responsible and ethical development of artificial intelligence.
Categories: Artificial Intelligence, Data Ethics, Synthetic Data, Machine Learning (ML), Data Privacy, Generative Models, AI Ethics and Governance, Future of Technology, Data Bias and Representation, Ethical AI Development
The following sources are cited as references used in research for this post:
Blum, A., & Dabbish, E. (2021). IoT Security Challenges: The Case of AI Botnets. Springer.
Goodfellow, I., Bengio, Y., & Courville, A. (2016). Deep Learning. MIT Press.
Kietzmann, J., Paschen, J., & Treen, E. (2018). Artificial Intelligence in Advertising: How AI can Improve Customer Relationships. Journal of Advertising Research.
O’Neill, C. (2016). Weapons of Math Destruction: How Big Data Increases Inequality and Threatens Democracy. Crown Publishing.
Silver, D., Schrittwieser, J., Simonyan, K., et al. (2017). Mastering Chess and Shogi by Self-Play with a General Reinforcement Learning Algorithm. Nature.
Glossary of terms used in this post:
Artificial Intelligence: The simulation of human intelligence in machines, enabling them to perform tasks such as learning, reasoning, and problem-solving.
Bias: Systematic and unfair influence in data or algorithms that can lead to prejudiced outcomes.
Carbon Footprint: The total amount of greenhouse gases, primarily carbon dioxide, emitted by an activity, product, or entity.
Data Augmentation: Techniques used to increase the size and diversity of a dataset by applying transformations to existing data.
Generative Adversarial Networks (GANs): A class of machine learning frameworks used to generate synthetic data by pitting two networks against each other.
Machine Learning (ML): A subset of AI focused on algorithms that learn from data to make predictions or decisions.
Privacy: The right of individuals to control their personal data and how it is used or shared.
Synthetic Data: Artificially generated data that imitates the characteristics of real-world data.
BearNetAI, LLC | © 2024, 2025 All Rights Reserved