AI Robots in Public Spaces
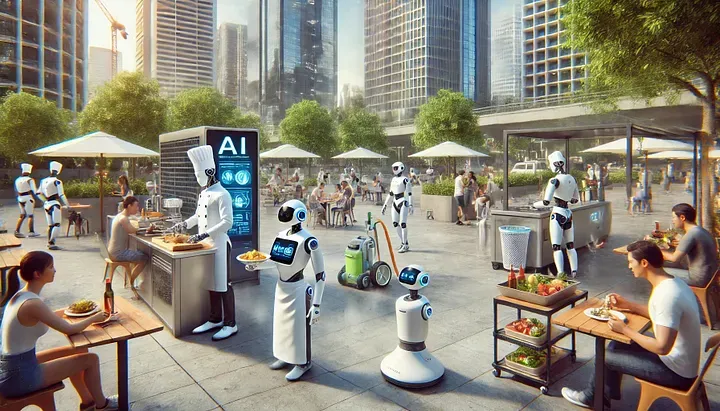
This week’s post is also available as a podcast if you prefer to listen on the go or enjoy an audio format:
Artificial intelligence has moved beyond science fiction into the fabric of our everyday world. One of the most visible signs of this shift is the growing presence of AI-powered robots in public areas. From preparing meals to maintaining cleanliness and engaging with people, these mechanical assistants are becoming familiar sights in our shared spaces. The combination of advances in generative AI and substantial financial backing from investors has quickened this development. While the progress is clear, these robots still face many obstacles in their essential functions, from moving through complex spaces to identifying objects accurately. As generative AI develops, it offers promising solutions to improve physical capabilities and social interactions, opening new possibilities across many industries.
Generative AI encompasses machine learning systems that can create original content — written material, visual elements, or movement patterns — based on their training data. This technology brings valuable benefits to robotics, especially in making machines more flexible and better at solving problems. Using generative AI, robots can practice and improve their tasks in virtual settings before working in real situations, making learning more efficient.
Consider a robot working in a kitchen: it can use generative AI to test different cooking methods and adjust recipes based on various preferences. Robots use this technology in cleaning applications to map out different scenarios with obstacles, learning the best ways to navigate and clean effectively. Companies like Boston Dynamics and Moley Robotics have already implemented these ideas, showing the real-world benefits of combining generative AI with robotics.
The path forward isn’t without its difficulties. Many tasks that humans do naturally still pose significant challenges for robots. Moving through busy public spaces requires constant adjustment as people and objects shift position — something robots find particularly difficult. They also struggle to handle everyday items in different shapes and sizes. Perhaps most challenging is understanding human communication in all its complexity, including subtle emotional cues and context-dependent meanings. These issues highlight the need for improvements in physical components and AI systems.
As engineers and developers address these technical challenges, AI robots find their place in various sectors, bringing a wave of optimism. Hotels test these machines to deliver items to rooms, maintain cleanliness, and welcome guests with personalized service. Theme parks employ them to manage visitor flow and provide guided tours. In healthcare settings, robots assist with patient care and logistics. Retail establishments use them to help customers find products and maintain stock levels. The potential for these robots to revolutionize these industries is fascinating.
We’re reshaping how people interact with machines in public spaces by bringing generative AI into robotics. Progress in language processing, visual recognition, and learning systems will enable robots to handle increasingly complex tasks with excellent skill and social awareness. This advancement could lead to lower operating costs and increased efficiency while creating new employment opportunities in AI development and system maintenance.
Integrating AI robots into public spaces raises important questions about privacy, employment changes, and the environmental impact of manufacturing these machines. However, the need for clear guidelines and regulations is paramount. These will help ensure this technological shift benefits everyone involved and reassure the audience about the responsible use of AI. We must carefully balance innovation with social responsibility, considering widespread robot deployment’s immediate benefits and long-term implications.
As we develop and refine AI robotics, we should focus on creating systems that complement rather than replace human capabilities. This emphasis on human values and an integral role in the process will make the audience feel appreciated. Success will depend on technical excellence and thoughtful implementation considering social impact, safety, and accessibility. The future of AI robots in public spaces holds excellent promise, but realizing that potential requires careful attention to both technical development and social integration.
Thank you for being a part of this fascinating journey.
BearNetAI. From Bytes to Insights. AI Simplified.
BearNetAI is a proud member of the Association for the Advancement of Artificial Intelligence (AAAI), and a signatory to the Asilomar AI Principles, committed to the responsible and ethical development of artificial intelligence.
Categories: AI Technology, Generative AI, Human-Robot Interaction, Public Spaces, Automation, Robotics, Technological Challenges
The following sources are cited as references used in research for this post:
Blum, A., & Dabbish, E. (2021). IoT Security Challenges: The Case of AI Botnets. Springer.
Brown, T., & Mann, B. (2020). Language Models Are Few-Shot Learners. OpenAI.
Ng, A. (2018). Machine Learning Yearning: Technical Strategy for AI Engineers in the Era of Deep Learning. deeplearning.ai.
Russell, S., & Norvig, P. (2020). Artificial Intelligence: A Modern Approach (4th ed.). Pearson.
Sutton, R. S., & Barto, A. G. (2018). Reinforcement Learning: An Introduction (2nd ed.). MIT Press.
Thrun, S., Burgard, W., & Fox, D. (2005). Probabilistic Robotics. MIT Press.
Glossary of terms used in this post:
Carbon Footprint: The total amount of greenhouse gases, primarily carbon dioxide, emitted by an activity, product, or entity.
Computer Vision: A field of AI that enables machines to interpret and process visual information from the world.
Generative AI: AI models capable of creating new content, such as text, images, or actions, based on input data.
Machine Learning: A subset of AI involving algorithms that improve automatically through experience and data analysis.
Natural Language Processing (NLP): A branch of AI focused on the interaction between computers and human languages.
Reinforcement Learning: A type of machine learning where agents learn by taking actions to maximize cumulative rewards in an environment.
Robotic Dexterity: The ability of robots to perform fine and precise movements, often resembling human hand and arm capabilities.
BearNetAI, LLC | © 2024, 2025 All Rights Reserved