Generative AI Challenges
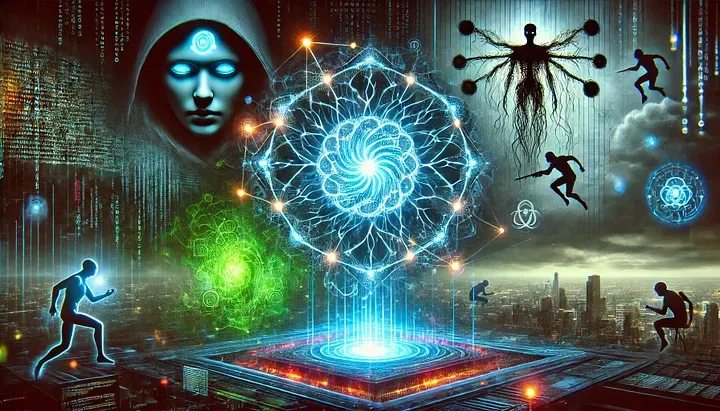
This week’s post is also available as a podcast if you prefer to listen on the go or enjoy an audio format:
Generative AI, an agent for a technological revolution, has permeated every facet of modern society. While these advanced systems offer unprecedented benefits, they open up new frontiers for security threats that demand immediate attention. As these technologies mature and become more accessible, security experts have sounded the alarm about their potential misuse in cyberattacks and fraudulent activities. The Federal Bureau of Investigation has already taken note, issuing stark warnings about criminals who exploit AI capabilities for phishing schemes and financial deception. The security landscape is further complicated by technical vulnerabilities such as adversarial machine learning and data manipulation, which pose direct threats to AI systems.
Modern generative AI systems, massive language models, and image generators can produce content closely resembling human output. These capabilities positively affect education, art, and customer service automation. However, this ability to create authentic-looking content also paves the way for malicious exploitation. The evolution of phishing attacks clearly demonstrates this risk — AI now empowers malicious actors to craft genuine and personal messages, rendering traditional security awareness less effective. These AI-enhanced phishing attempts succeed by analyzing communication patterns and tailoring messages that resonate with specific individuals or groups.
The financial industry is under increasing pressure from AI-enabled fraud schemes. Modern AI tools can fabricate synthetic personas by blending real and artificial information, pushing the boundaries of current identity verification methods. Financial institutions are now grappling with AI systems that can mimic standard transaction patterns, making it more difficult to detect fraudulent activities such as account theft and illegal money movement. These challenges require innovative approaches from banks, regulatory bodies, and law enforcement agencies.
The security of AI systems faces internal threats through adversarial machine learning techniques. These attacks involve subtle modifications to input data that cause AI systems to fail in their tasks. For example, minor image changes can fool recognition systems, while altered audio signals can mislead voice-activated devices. These vulnerabilities raise serious questions about the reliability of AI in critical applications like security systems and automated transportation.
The integrity of AI systems also faces threats from training data manipulation. When malicious actors insert false or misleading information into training datasets, they can alter how AI systems learn and make decisions. This attack affects every sector that relies on AI-driven decision-making, from medical diagnostics to financial forecasting.
As AI technology becomes more accessible through open-source models and simple interfaces, more individuals gain the ability to deploy these tools — for good and bad. While this access drives innovation, it also reduces barriers for those seeking to misuse the technology. Finding solutions requires a careful balance between promoting beneficial use and preventing harm.
The technical community actively develops solutions to protect AI systems from attacks. Current research focuses on creating AI models that maintain accuracy even when faced with deceptive inputs. Scientists implement new training methods that expose AI systems to potential attacks during development, helping them build natural defenses. Modern security systems now include continuous monitoring capabilities to detect and stop suspicious AI behavior.
Effective oversight of generative AI requires thoughtful regulation. Government agencies must create standards for responsible AI development and use while ensuring companies maintain transparency about their AI systems’ capabilities and limitations. Given the global nature of cyber threats, countries must work together to create consistent approaches to AI security.
Teaching people about AI-related threats is as important as technical solutions. The public needs practical knowledge about identifying AI-generated scams and verifying digital content’s authenticity. Creating informed users who understand AI’s potential and risks helps build a more secure digital environment.
The future of generative AI security depends on how well we adapt to evolving challenges. Success requires ongoing innovation in protective measures, smart regulation, and public education. By addressing security concerns now, we can help ensure that AI’s benefits to society outweigh its risks. The path forward demands constant vigilance and adaptation as AI capabilities and potential threats evolve.
Thank you for being a part of this fascinating journey.
BearNetAI. From Bytes to Insights. AI Simplified.
BearNetAI is a proud member of the Association for the Advancement of Artificial Intelligence (AAAI), and a signatory to the Asilomar AI Principles, committed to the responsible and ethical development of artificial intelligence.
Categories: Cybersecurity, Artificial Intelligence, Generative AI, Machine Learning, Digital Fraud
The following sources are cited as references used in research for this post:
Blum, A., & Dabbish, E. (2021). IoT Security Challenges: The Case of AI Botnets. Springer.
Goodfellow, I., Bengio, Y., & Courville, A. (2016). Deep Learning. MIT Press.
Hoffman, D. (2020). Cybersecurity and Cyberwar: What Everyone Needs to Know. Oxford University Press.
Ng, A. Y., & Jordan, M. I. (2001). On Discriminative vs. Generative Classifiers: A Comparison of Logistic Regression and Naive Bayes. Advances in Neural Information Processing Systems.
Vincent, J. (2018). AI Superpowers: China, Silicon Valley, and the New World Order. Houghton Mifflin Harcourt.
Glossary of terms used in this post:
Adversarial Attack: A technique used to deceive AI models by subtly modifying inputs to produce incorrect outputs.
Adversarial Training: Enhancing AI resilience by exposing models to adversarial examples during development.
Data Poisoning: Injecting malicious or misleading data into training datasets to influence AI behavior.
Generative AI: Artificial intelligence systems capable of creating new content, such as text, images, or audio, based on input data.
Phishing: A cyberattack technique that uses deceptive communication to steal sensitive information.
Synthetic Identity: A fictitious identity created by combining real and fake information, often used for fraud.
BearNetAI, LLC | © 2024, 2025 All Rights Reserved