How AI Assistants Learn from Your Conversations
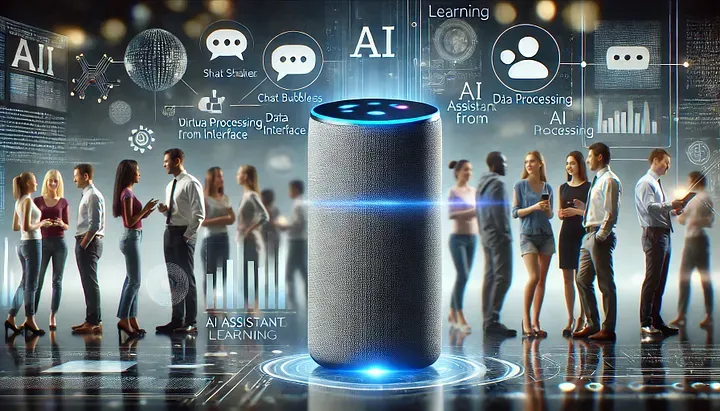
Artificial intelligence has become increasingly integrated into our daily lives in recent years, with AI assistants like ChatGPT, Siri, Alexa, and Google Gemini playing pivotal roles. These AI assistants, powered by complex algorithms and vast datasets, can engage in natural conversations, provide helpful information, and even learn from interactions to improve their performance over time. Understanding how AI assistants learn from conversations is critical to appreciating the technology’s potential and bright future.
AI assistants rely on machine learning (ML) and natural language processing (NLP) to understand, process, and generate human language. NLP is a branch of AI that focuses on the interaction between computers and human language, enabling machines to interpret, understand, and respond to text or speech meaningfully and contextually appropriately.
When you interact with an AI assistant, your inputs — whether typed or spoken — are first analyzed using NLP techniques. The AI breaks down your sentence structure, identifies critical components such as nouns, verbs, and objects, and interprets the overall meaning. This process is supported by large language models, like GPT-4, which have been trained on vast datasets containing diverse examples of human language. These models draw on this training to generate responses that align with your query’s context and intent.
One of the most intriguing aspects of AI assistants is their remarkable adaptability. They can learn and evolve through interaction, primarily through supervised learning and reinforcement learning.
In this method, the AI model is trained on a labeled dataset, where each input is paired with a correct output. For example, if a user asks, “What is the weather today?” the correct output might be a detailed weather report. The AI learns from these examples, understanding which types of responses are appropriate for different queries. Over time, the assistant becomes more adept at generating accurate and relevant answers based on user input.
Reinforcement learning is another way the AI learns from feedback received during interactions. After generating a response, the AI may receive input directly from the user or inferred from the user’s continued engagement or disengagement. Positive feedback (such as a user saying “Thank you” or asking follow-up questions) reinforces the AI’s behavior. In contrast, negative feedback (like rephrasing the question or expressing dissatisfaction) signals that the response is suboptimal. Through this trial-and-error process, the AI fine-tunes its responses to better meet user expectations.
As AI assistants interact with users over time, they recognize patterns and preferences, allowing for a more personalized experience. This personalization is achieved through contextual learning, where the AI remembers specific details from past conversations to enhance future interactions.
For instance, if you frequently ask an AI assistant for news updates on specific topics like artificial intelligence or international politics, the assistant may prioritize these subjects in future interactions. Similarly, the AI can adapt its responses accordingly if you prefer receiving summaries rather than detailed reports.
However, it’s important to note that the extent of personalization can vary depending on the AI assistant’s design and privacy settings. Some AI systems may retain more detailed contextual information to provide a highly tailored experience, while others may prioritize user privacy and limit the amount of personal data stored.
While AI assistants’ learning capabilities are impressive, they are not without challenges and ethical considerations. One significant challenge is ensuring that AI assistants understand and respect user privacy. As these systems learn from interactions, they collect and process large amounts of data, raising concerns about how it is stored, used, and shared. Developers must implement robust privacy safeguards and give users control over their data.
Another challenge is mitigating biases in AI models. Since AI assistants learn from data that reflects human language and behavior, they can inadvertently absorb and perpetuate biases in the data. This can lead to biased or unfair responses, which can have real-world consequences. Ongoing efforts are needed to identify and address biases in AI systems to ensure that they provide fair and equitable interactions.
Finally, there is the question of transparency. Users should be aware of how AI assistants learn from their conversations and the implications of this learning. Transparent communication about data usage, personalization, and the limitations of AI systems is essential for building trust between users and AI technologies.
AI assistants have come a long way in learning from conversations, thanks to advancements in machine learning, natural language processing, and reinforcement learning. These technologies enable AI assistants to engage in meaningful interactions, adapt to user preferences, and provide increasingly personalized experiences. However, addressing the challenges and ethical considerations associated with data privacy, bias, and transparency is essential as AI evolves. By doing so, we can harness the full potential of AI assistants while ensuring that they operate in a manner that is both beneficial and respectful to users.
Join Us Towards a Greater Understanding of AI
By following us and sharing our content, you’re not just spreading awareness but also playing a crucial role in demystifying AI. Your insights, questions, and suggestions make this community vibrant and engaging. We’re eager to hear your thoughts on topics you’re curious about or wish to delve deeper into. Together, we can make AI accessible and engaging for everyone. Let’s continue this journey towards a better understanding of AI. Please share your thoughts with us via email: marty@bearnetai.com, and don’t forget to follow and share BearNetAI with others who might also benefit from it. Your support makes all the difference.
Thank you for being a part of this fascinating journey.
BearNetAI. From Bytes to Insights. AI Simplified.
Categories: Artificial Intelligence, Technology and Innovation, Machine Learning, Ethics in AI, Privacy and Data Security, Personalization in Technology, Human-Computer Interaction
The following sources are cited as references used in research for this BLOG post:
Artificial Intelligence: A Guide for Thinking Humans by Melanie Mitchell
Human Compatible: Artificial Intelligence and the Problem of Control by Stuart Russell
Deep Learning by Ian Goodfellow, Yoshua Bengio, and Aaron Courville
Weapons of Math Destruction: How Big Data Increases Inequality and Threatens Democracy by Cathy O’Neil
The Age of Surveillance Capitalism: The Fight for a Human Future at the New Frontier of Power by Shoshana Zuboff
You Look Like a Thing and I Love You: How AI Works and Why It’s Making the World a Weirder Place by Janelle Shane
The Fourth Industrial Revolution by Klaus Schwab
© 2024 BearNetAI LLC