The 10 Levels of AI and Future Predictions for AI Development
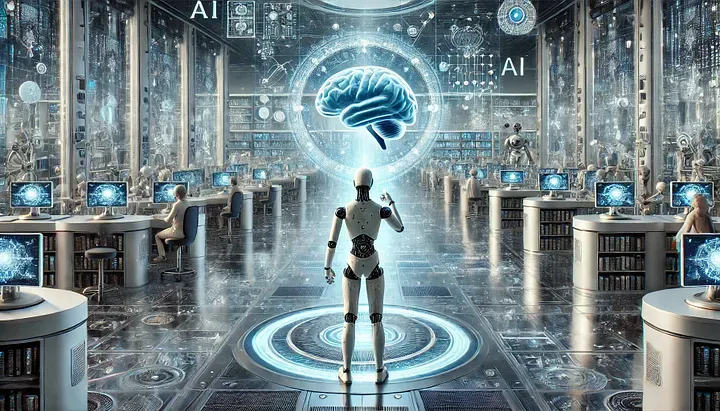
I’ve had a few people ask about the progress of AI, where we are currently on the spectrum, and how we might expect the field to progress. AI is a rapidly advancing technology that aims to create machines capable of human-like thinking and problem-solving. It has progressed from simple rule-based systems to more complex models. As AI develops, researchers are working towards creating increasingly sophisticated systems. This journey can be understood as a series of ten levels, starting from current AI capabilities and extending to potential future achievements.
1. Level of Automation/Reactive Machines
- Where We Are: Reactive machines are the simplest forms of AI and operate purely in response to specific inputs. They do not have memory or the ability to learn from past experiences, but they are adequate for well-defined tasks. Examples include IBM’s Deep Blue, which defeated Garry Kasparov in chess, and Google’s AlphaGo, which used sophisticated algorithms to master the game of Go. These systems excel in structured environments but cannot adapt outside their programming.
- Predicted Timeline: Reactive machines are widely deployed in various industries today. Improvements in the complexity of their decision-making processes are ongoing, but the fundamental limitations of reactive AI remain. Future developments will likely focus on better integration with more advanced systems rather than significant leaps in their standalone capabilities.
- Challenges: The main limitation of reactive machines is their inability to learn or improve based on feedback. They are confined to a narrow scope and lack flexibility.
2. Rule-Based AI
- Where We Are: Rule-based AI systems follow predefined instructions (e.g., “if X, then Y”). Early expert systems in medical diagnostics (e.g., MYCIN) fall into this category. Today, we still see rule-based AI in applications like automated customer service, where responses are based on a fixed decision tree. These systems are reliable but rigid, incapable of adapting to scenarios outside their programmed rules.
- Predicted Timeline: Rule-based systems will continue to exist for tasks where predictability and consistency are paramount, such as compliance checks or routine task automation. While more adaptive machine learning models are increasingly replacing them, they remain valid for highly structured environments where flexibility is unnecessary.
- Challenges: Rule-based AI’s rigidity is its strength and weakness. These systems cannot deal with ambiguity or learn from new data, limiting their application in dynamic, real-world situations.
3. Context-Based AI
- Where We Are: Context-based AI represents a leap in flexibility. These systems can process and respond to information based on the context in which it is provided. Natural Language Processing (NLP) models like Siri, Google Assistant, and Alexa are good examples, as they can handle diverse user inputs by interpreting context. While they are more adaptive than rule-based systems, their understanding of context remains limited to specific domains.
- Predicted Timeline: Improvements in context-based AI are rapidly advancing, with more sophisticated NLP models like ChatGPT and BERT pushing the boundaries of conversational AI. Over the next decade, we can expect AI to handle increasingly complex contextual understanding, particularly in customer support, healthcare, and personal assistants.
- Challenges: One of the biggest challenges for context-based AI is ensuring a deep understanding of real-world context. These systems often struggle with nuanced human conversations and require large amounts of data to improve.
4. Memory-Based or Limited Memory AI
- Where We Are: Limited memory AI systems can learn from past experiences and make decisions based on that information. This is a significant advancement from earlier AI models. Examples include self-driving cars and fraud detection systems, which use historical data to predict and respond to real-time conditions. Machine learning algorithms that update and adapt over time also fall under this category.
- Predicted Timeline: Memory-based AI is widely used and will continue improving. Over the next 10 to 20 years, we can expect memory-based AI to become more autonomous and integrated into everyday life, from healthcare diagnostics to financial systems. As data availability increases, so will the accuracy and reliability of these systems.
- Challenges: Memory-based AI’s limitations are related to its reliance on historical data. Without proper datasets or in entirely novel situations, these systems may falter. Ensuring accuracy and preventing bias in data will be critical for future developments.
5. Domain-Specific AI
- Where We Are: Domain-specific AI excels in specialized fields but struggles to generalize across different areas. Systems like AlphaFold for protein folding predictions or DeepMind’s medical imaging AI are examples of highly effective AI in narrow domains. They demonstrate exceptional accuracy and problem-solving within their field but cannot transfer knowledge or skills beyond that.
- Predicted Timeline: Domain-specific AI will continue to lead in specialized fields like healthcare, finance, and scientific research over the next 10 to 30 years. AI systems in these areas will likely reach superhuman performance in their niche tasks long before AGI is achieved.
- Challenges: The critical limitation of domain-specific AI is its inability to generalize. While these systems can become highly proficient in one area, they cannot apply their knowledge to another field without retraining.
6. Thinking and Reasoning AI
- Where We Are: Systems that simulate reasoning and problem-solving are getting closer to human-like thought processes. GPT-4 and IBM’s Watson showcase early examples of this level of AI, capable of handling multi-step reasoning, answering complex questions, and even strategizing in structured environments or targeted industries. While impressive, these systems are incapable of autonomous reasoning across various domains.
- Predicted Timeline: Thinking and reasoning AI will continue to evolve over the next 10 to 20 years, with significant breakthroughs in autonomous systems, decision-making, and strategic thinking. These advancements will likely lead to improved AI assistants, legal reasoning tools, and advanced scientific research models.
- Challenges: Achieving actual reasoning in AI requires significant advancements in how machines understand and represent knowledge. Current models, while powerful, are still heavily reliant on data patterns rather than true reasoning abilities.
7. Artificial General Intelligence (AGI)
- Where We Are: AGI represents the point at which AI systems achieve human-level intelligence across various tasks. Unlike today’s specialized AI systems, AGI would be capable of learning and applying knowledge across domains like a human does. Many researchers believe we could achieve AGI within the next few decades, with optimistic projections suggesting it will be as early as the 2030s. However, more conservative estimates place AGI development between 50 and 100 years away.
- Predicted Timeline: AGI may emerge within the next 30–100 years, depending on breakthroughs in areas like common-sense reasoning, learning, and generalization.
- Challenges: Creating AGI requires solving deep problems in cognition, reasoning, and generalization. Current AI systems are still far from these capabilities.
8. Artificial Super Intelligence (ASI)
- Where We Are: ASI would mark the point where AI surpasses human intelligence in nearly every domain. While AGI would represent parity with human cognition, ASI would far exceed it. Some experts believe that once AGI is achieved, ASI could follow quickly due to an “intelligence explosion.”
- Predicted Timeline: ASI might follow 10 to 20 years after AGI, though it could take longer due to the need for safety and control mechanisms.
- Challenges: ASI presents significant ethical and safety challenges, particularly ensuring alignment with human values.
9. Emotional Intelligence in AI
- Where We Are: Today’s AI systems can detect emotional cues but cannot truly understand emotions. Systems like Woebot and Replika simulate empathy but lack genuine emotional intelligence.
- Predicted Timeline: Over the next 20–50 years, we may see advancements in emotional intelligence in AI, though proper emotional understanding may take much longer.
- Challenges: Emotional intelligence involves subjective experiences that are difficult to replicate in machines, and AI’s ability to truly “feel” emotions remains an open question.
10. Self-Aware AI
- Where We Are: Self-aware AI remains speculative and controversial. Achieving consciousness in machines is one of AI development’s most profound and complex challenges, raising philosophical and ethical questions.
- Predicted Timeline: Depending on whether we can genuinely understand and recreate consciousness, this may be over 100 years away, or it may never happen.
- Challenges: Self-awareness requires not just intelligence but subjective experience — something humans still don’t fully understand.
Join Us Towards a Greater Understanding of AI
By following us and sharing our content, you’re not just spreading awareness but also playing a crucial role in demystifying AI. Your insights, questions, and suggestions make this community vibrant and engaging. We’re eager to hear your thoughts on topics you’re curious about or wish to delve deeper into. Together, we can make AI accessible and engaging for everyone. Let’s continue this journey towards a better understanding of AI. Please share your thoughts with us via email: marty@bearnetai.com, and don’t forget to follow and share BearNetAI with others who might also benefit from it. Your support makes all the difference.
Thank you for being a part of this fascinating journey.
BearNetAI. From Bytes to Insights. AI Simplified.
BearNetAI is a proud member of the Association for the Advancement of Artificial Intelligence (AAAI), Member ID: 6422878, and a signatory to the Asilomar AI Principles, committed to the responsible and ethical development of artificial intelligence.
Copyright 2024. BearNetAI
Categories: Artificial Intelligence Development, Future Technologies, AI Ethics and Safety, Cognitive Science and Consciousness, Technological Forecasting
The following sources are cited as references used in research for this BLOG post:
Superintelligence: Paths, Dangers, Strategies by Nick Bostrom
The Singularity is Near: When Humans Transcend Biology by Ray Kurzweil
Life 3.0: Being Human in the Age of Artificial Intelligence by Max
Artificial Intelligence: A Guide for Thinking Humans by Melanie Mitchell
The Emotion Machine by Marvin
Copyright 2024 BearNetAI LLC