The Impact of AI on Pricing Efficiency vs. Discrimination
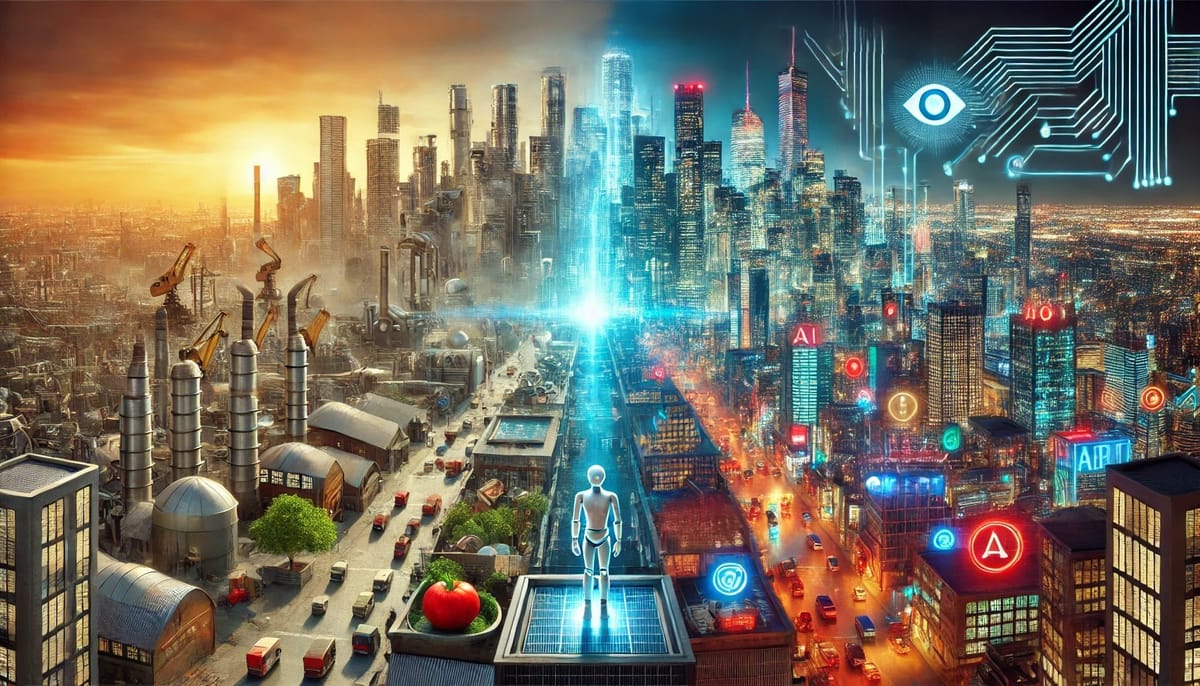
This post is also available as a podcast if you prefer to listen on the go or enjoy an audio format:
Artificial Intelligence is fundamentally transforming global economies at an unprecedented pace, creating ripples across every sector, from manufacturing to retail. While AI promises efficiency gains, cost reductions, and productivity boosts, it presents an economic paradox. On the one hand, these technological advancements should translate into lower prices for consumers through streamlined operations and optimized supply chains. On the other hand, the growing concentration of AI capabilities within powerful corporate entities raises legitimate concerns about whether these cost savings will reach consumers or bolster corporate profits.
This tension between technological efficiency and corporate power sits at the heart of our economic future. As AI continues evolving and penetrating our financial systems, we must examine the promise and peril it presents for consumers, workers, and society. This post explores the dual nature of AI's economic impact, examining how it simultaneously offers pathways to greater affordability while potentially reinforcing monopolistic tendencies that could undermine those benefits.
Across factory floors worldwide, AI-powered robotics and automated systems dramatically improve manufacturing efficiency. These systems work with precision and consistency that exceed human capabilities, reducing labor costs while minimizing material waste and quality control issues. Automotive factories represent this transformation in action—their AI-driven production lines enable vehicles to be manufactured at increasingly competitive prices compared to traditional automotive manufacturing methods.
Similar transformations are unfolding in electronics, pharmaceuticals, and consumer goods production. AI systems continuously learn from operational data, identifying inefficiencies and suggesting improvements that human managers might overlook. This relentless optimization creates a virtuous cycle of cost reduction that, under ideal market conditions, should result in more affordable products for consumers.
The movement of products from raw materials to consumers' hands has historically been fraught with inefficiencies—overstocking, shortages, spoilage, and transportation delays. AI is fundamentally reimagining this landscape by predicting demand patterns with remarkable accuracy, streamlining distribution networks, and ensuring products reach their destinations through optimal routes.
Companies like Amazon exemplify this supply chain revolution. Their AI algorithms orchestrate intricate warehouse operations, determining everything from inventory placement to picking routes. These systems predict consumer demand patterns for specific neighborhoods and periods, enabling preemptive stocking of fulfillment centers. The result is faster delivery for consumers and significantly reduced operational costs—fewer warehouses can serve more customers with less inventory sitting idle.
Similarly, global shipping companies now employ AI to optimize container placement, route planning, and port scheduling, reducing fuel consumption and transit times. These efficiencies ripple through the entire supply chain, lowering the final cost of goods across retail sectors.
The traditional retail model operated on broad assumptions about consumer preferences, leading to substantial waste in advertising, inventory, and customer acquisition. AI has fundamentally altered this equation by enabling unprecedented personalization on a large scale. E-commerce platforms now analyze browsing patterns, purchase history, and contextual factors to create uniquely tailored shopping experiences for individual consumers.
This personalization dramatically reduces marketing inefficiencies. Rather than broad campaigns with low conversion rates, retailers can precisely target consumers with products they're genuinely likely to purchase. This intelligence also extends to inventory management—stores stock precisely what their specific customer base desires, reducing markdowns and waste.
Dynamic pricing represents the most controversial aspect of retail AI. These systems adjust prices in real-time based on numerous factors, including demand patterns, competitor pricing, inventory levels, and individual consumer behavior. While this raises ethical concerns about fairness, it can benefit consumers during periods of excess inventory when algorithms lower prices to stimulate demand.
The production and distribution of goods depend fundamentally on energy inputs, which traditionally represent significant business cost centers. AI systems are revolutionizing energy management through intelligent monitoring and control systems that drastically reduce consumption.
Google's implementation of DeepMind AI to manage data center cooling systems exemplifies this potential. By analyzing thousands of sensors and continuously optimizing cooling parameters, the system reduced energy consumption by 40%—a remarkable achievement considering the enormous energy footprint of global data infrastructure.
Similar innovations appear across manufacturing, with AI systems monitoring production lines and adjusting parameters in real-time to minimize energy usage while maintaining output quality. These savings represent significant operational cost reductions that corporations could pass on through more competitive pricing.
While AI undoubtedly reduces operational costs across numerous industries, a fundamental question emerges: will these savings benefit consumers through lower prices, or will they primarily increase corporate profit margins? Historical precedent gives reason for concern. Companies tend to capture efficiency gains as profit in sectors where competition is limited rather than passing savings to consumers.
This tendency becomes particularly problematic as AI accelerates market concentration. Companies with superior AI capabilities enjoy compounding advantages—better customer insights, more efficient operations, and enhanced ability to predict market trends. These advantages can create feedback loops that further reinforce their market dominance, potentially resulting in monopolistic or oligopolistic market structures where price competition is minimal.
The technology sector already demonstrates this pattern. Despite enormous efficiency gains through AI and automation, consumer prices for many digital services haven't fallen proportionally. Instead, profit margins for dominant technology firms have reached historic highs, suggesting that efficiency gains primarily benefit shareholders rather than consumers.
While dynamic pricing algorithms can occasionally benefit consumers, they enable sophisticated price discrimination practices that may harm consumer welfare. These systems can analyze vast amounts of behavioral data to determine how much different consumers are willing to pay for identical products or services.
The airline industry offers a troubling preview of how this might evolve. Identical seats on the same flight can vary dramatically in price based on purchase timing, browser cookies, search history, and numerous other factors. This level of price discrimination becomes increasingly sophisticated with AI, potentially creating a marketplace where each consumer sees uniquely calibrated prices designed to extract maximum revenue.
More concerning still is the potential for algorithmic bias in these systems. Suppose pricing algorithms incorporate factors that correlate with protected characteristics like race, gender, or socioeconomic status. In that case, they may inadvertently perpetuate or amplify existing social inequalities, charging higher prices to disadvantaged groups.
The same efficiency gains that potentially lower consumer prices also threaten millions of jobs across manufacturing, retail, customer service, and logistics. While economists debate the long-term employment effects of automation, the short-term disruption is undeniable. Warehouse workers, cashiers, call center representatives, and truck drivers face immediate displacement as AI systems increasingly perform their tasks more efficiently.
This displacement creates a paradoxical scenario: consumer goods might become more affordable precisely as many consumers lose their income sources. Without thoughtful transition policies, the efficiencies that drive price reductions could simultaneously fuel economic inequality and reduce overall purchasing power for significant segments of society.
Even for those who maintain employment, AI potentially negatively affects wages in many sectors. As automation reduces demand for specific skills, workers in those fields face diminished bargaining power. This wage suppression could offset any benefits from reduced consumer prices, resulting in a net loss of purchasing power for many households.
While AI may reduce production costs for many consumer goods, it paradoxically may increase prices for scarce resources and premium products. As essential goods become more affordable, consumer attention and status-seeking naturally shift toward differentiators—unique experiences, artisanal products, prime real estate, and luxury goods.
Land represents the most obvious example of this dynamic. No amount of AI efficiency can create more beachfront property or downtown real estate in desirable cities. As overall prosperity increases from AI-driven efficiency, competition for these inherently limited resources intensifies, driving prices upward for assets that cannot be replicated through technology.
This could create a bifurcated economy where mass-produced goods become increasingly affordable while uniqueness becomes increasingly expensive. Such a scenario might deepen social stratification, with access to certain status goods and experiences becoming markers of class division rather than purely reflections of personal preference.
The AI systems driving pricing efficiency depend on unprecedented consumer data collection and analysis levels. This "surveillance capitalism" model raises profound questions about privacy, autonomy, and the commodification of human behavior. Consumers often receive AI-enabled price benefits without understanding the value of the data they surrender in exchange.
As AI pricing systems grow more sophisticated, they increasingly rely on intimate knowledge of consumer psychology and behavior patterns. This creates uncomfortable power dynamics where corporations understand consumers better than consumers understand themselves, potentially enabling manipulation rather than simply meeting needs efficiently.
Moreover, the vast data infrastructure required to power these AI systems carries environmental and social costs. Server farms consume enormous energy resources, and the necessary minerals for computing hardware often come from regions with questionable labor and environmental standards. These externalized costs rarely factor into consumer prices but represent actual social expenses of our AI-driven economy.
Traditional regulatory approaches struggle to address the challenges of AI-driven pricing and market concentration. Addressing this gap requires innovative regulatory frameworks explicitly designed for algorithmic markets.
Antitrust enforcement must evolve beyond simplistic measures like market share to consider data advantages, network effects, and algorithmic coordination. Regulators need technical capacity to audit pricing algorithms for anti-competitive behavior and discriminatory outcomes. This might require algorithm transparency, particularly for essential goods and services where discriminatory pricing could cause significant harm.
International coordination becomes increasingly important as AI systems operate across national boundaries. Regional approaches like the European Union's Digital Markets Act provide potential models for preventing market domination while still encouraging innovation. Creating global standards for algorithmic fairness and competition could help prevent regulatory arbitrage, where companies relocate to jurisdictions with minimal oversight.
Beyond formal regulation, developing widely accepted ethical standards for AI pricing practices could help ensure these systems benefit consumers rather than exploit them. Organizations have already begun developing AI fairness frameworks that could be applied specifically to pricing algorithms.
These standards include requirements for explainability (ensuring consumers understand why they're seeing specific prices), fairness (preventing discriminatory outcomes), and equity (ensuring AI benefits are broadly distributed). Companies could be encouraged to certify compliance with these standards through independent audits, creating market incentives for ethical AI deployment.
Some industries might develop sector-specific AI governance models adapted to their unique characteristics. Healthcare, housing, and education—areas where algorithmic pricing could have profound social impacts—might require particularly stringent standards ensuring that efficiency gains translate to broader affordability rather than increased profits.
As pricing becomes increasingly algorithmic, consumers need new tools to navigate this complex landscape. AI-powered consumer protection applications could analyze platform pricing patterns, alert consumers to potential discrimination, and recommend optimal purchase timing based on algorithmic predictions.
Price transparency platforms aggregate consumer data to identify unfair practices or excessive price discrimination. These tools could function similarly to credit score monitoring services, helping individuals understand how they're being categorized and priced by various algorithms and suggesting actions to improve their algorithmic profile.
Education represents another critical component. Developing public literacy around how AI pricing works would help consumers make more informed choices and recognize potentially exploitative practices. This knowledge would create market pressure for companies to deploy AI pricing in ways that genuinely benefit consumers rather than extract maximum revenue.
The efficiency gains driving potential price reductions also displace workers across numerous industries. Addressing this displacement requires comprehensive workforce transition strategies operating at multiple levels.
Universal Basic Income experiments provide one potential approach, ensuring that efficiency gains from AI are broadly shared rather than concentrated among technology owners. Several countries and cities have piloted UBI programs with promising results, suggesting viable models for larger-scale implementation as AI accelerates workforce disruption.
Equally important are education and reskilling initiatives that help workers adapt to an AI-transformed economy. The most effective approaches combine traditional educational institutions with emerging AI-powered learning platforms that provide personalized skill development pathways. These initiatives require substantial investment but are essential to ensuring AI benefits extend beyond consumer prices, including employment opportunities.
Industry-specific transition programs developed through collaboration between employers, workers, educational institutions, and governments could provide targeted support for sectors facing significant AI disruption. These coordinated approaches can help entire communities navigate technological transitions rather than leaving individual workers to manage these shifts alone.
Perhaps the most potent strategy for ensuring AI benefits consumers involves democratizing access to technology itself. Open-source AI initiatives make advanced capabilities available to smaller businesses, cooperatives, and public institutions rather than concentrating them within large corporations.
Community-owned AI cooperatives offer another promising model. These organizations could develop and deploy AI pricing systems with explicit commitments to consumer benefit rather than profit maximization. Such models might be valuable in essential sectors like housing, healthcare, and food distribution.
Public research institutions can also play a crucial role in democratizing AI benefits. Government-funded research that remains in the public domain ensures that fundamental algorithmic advances benefit society broadly rather than creating monopolistic advantages for individual firms. This approach has a historical precedent in many technological revolutions, where public investment created platforms for broader innovation.
The impact of AI on consumer prices reflects a broader tension within technological progress—the gap between theoretical potential and actual distribution of benefits. While AI undoubtedly creates enormous efficiency gains capable of reducing costs, these benefits won't automatically flow to consumers without a deliberate effort to shape market structures, incentives, and governance frameworks.
History offers both cautionary tales and inspiring examples. Previous technological revolutions sometimes enhanced monopoly power and inequality, while in other cases, they dramatically improved living standards for ordinary people. The difference often hinged on social choices, policy frameworks, and power distributions that determined how efficiency gains were allocated.
The AI economic transition remains in its early stages, with much of its trajectory still subject to human agency and collective choice. By developing thoughtful governance models, empowering consumers, supporting workforce transitions, and democratizing access to AI, we can increase the likelihood that algorithmic efficiency translates to genuine affordability rather than concentrated wealth.
The fundamental question isn't whether AI will reduce costs, as it already is doing across numerous sectors. The real question is who will capture these gains and how will they reshape our economic landscape. Answering this question thoughtfully may be one of the most consequential challenges of our era.
Books by the Author:

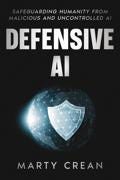
Categories
AI and Economics, Corporate Ethics, AI Governance, Surveillance and Privacy, Economic Policy
Citations:
Acemoglu, D., & Restrepo, P. (2020). Automation and the Future of Work. Journal of Economic Perspectives.
Blum, A., & Dabbish, E. (2021). IoT Security Challenges: The Case of AI Botnets. Springer.
Brynjolfsson, E., & McAfee, A. (2014). The Second Machine Age: Work, Progress, and Prosperity in a Time of Brilliant Technologies. W.W. Norton & Company.
Ford, M. (2015). Rise of the Robots: Technology and the Threat of a Jobless Future. Basic Books.
Zuboff, S. (2019). The Age of Surveillance Capitalism: The Fight for a Human Future at the New Frontier of Power. PublicAffairs.
BearNetAI, LLC | © 2024, 2025 All Rights Reserved
LinkedIn BlueskySignal: bearnetai.28