The Quest for Common Sense in Artificial Intelligence
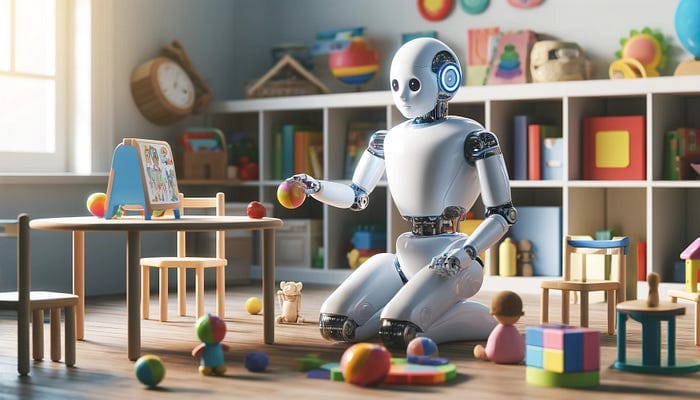
Common sense, often referred to as the ‘dark matter’ of artificial intelligence, is the unspoken and usually unconscious knowledge we assume others also possess, making it a cornerstone of human interaction and societal function. In the ever-evolving field of AI, the quest to instill machines with ‘common sense’ stands as one of the most intriguing and challenging frontiers. This short essay delves into the importance of common sense in AI, the challenges associated with this effort, and the potential paths forward, offering a glimpse into the promising future of AI research.
Common sense is not just a desirable trait for AI, it is crucial. It underpins the ability of AI to operate effectively and safely in human environments. AI systems, especially those interacting directly with people or performing tasks in dynamic, unstructured settings, must be able to make decisions that align with human expectations and norms. For example, an AI personal assistant should understand that if a user asks for a wake-up call at ‘dawn,’ this refers to the time around sunrise, which varies by location and date rather than a fixed hour. This underscores the urgency and significance of instilling AI with common sense.
Moreover, common sense enables AI to handle human language and behavior’s nuanced and often ambiguous nature. This capability is essential for natural language processing tasks such as conversation and text interpretation and makes AI systems more robust against errors and unexpected situations. Without common sense, AI might misinterpret instructions, make inappropriate decisions, or fail to recognize when it is being manipulated or encountering misleading information.
Developing AI with common sense is a formidable task, given the inherent complexity of human knowledge and the subtlety with which it is applied. Current AI systems, including sophisticated models like large language models (LLMs), excel in specific domains where they can leverage vast training data. However, these systems often struggle with simple intuitive tasks that any human could easily handle, such as understanding basic physical principles or social interactions that are not explicitly taught but are learned implicitly over years of human experience. This complexity underscores the depth of the challenge we face in this area of research.
One major challenge is the “data problem.” AI systems learn from data; common sense knowledge is vast, varied, and often not explicitly documented. Much of common sense constitutes implicit knowledge — unspoken understanding or skills that people intuitively know without formal education. Capturing this breadth of understood knowledge in a form that AI can learn from is an ongoing challenge.
The current approach to AI development heavily relies on statistical learning models that predict outputs based on patterns seen in training data. While powerful, these models do not inherently understand the causal relationships or underlying principles that guide common sense reasoning. They often produce errors when faced with scenarios that deviate from their training data, revealing a lack of deep comprehension.
Addressing the common sense challenge in AI requires innovative approaches beyond traditional data-driven methods. One promising direction is integrating machine learning with symbolic AI, which involves using explicit, rule-based systems to process and reason about the world. This hybrid approach can leverage the strengths of both methodologies: the adaptability and learning capabilities of machine learning and the structured reasoning and rule-following capabilities of symbolic AI. These potential solutions offer a reassuring glimpse into the future of AI research.
Another approach is to develop and deploy more interactive and experiential learning environments for AI, akin to how humans learn. By interacting with the world and receiving feedback, AI systems can better understand how things work, much like a child learns through play and interaction. This method could involve virtual or augmented reality systems where AI can safely experiment and learn from various simulated experiences.
Researchers are also exploring the creation of extensive common sense knowledge databases that AI systems can query and use. These databases would include a vast array of human knowledge, from physical laws to social customs, and be structured so that AI can understand and apply when needed. Efforts to make these resources openly available and crowd-sourced could significantly enhance their richness and accuracy.
The effort to instill AI with common sense is not just a technical challenge; it’s a necessary step toward making AI truly integrated and valuable in everyday human environments. As AI continues to evolve, the focus on developing common sense capabilities will be crucial for ensuring that these technologies can serve humanity effectively, safely, and ethically. Through innovative approaches, collaborative research, and a deeper understanding of human knowledge and AI capabilities, the goal of common sense AI, once seen as a distant dream, is increasingly within reach.
As the old saying goes, “Common sense isn’t so common.”
Join Us Towards a Greater Understanding of AI
We hope you found insights and value in this post. If so, we invite you to become a more integral part of our community. By following us and sharing our content, you help spread awareness and foster a more informed and thoughtful conversation about the future of AI. Your voice matters, and we’re eager to hear your thoughts, questions, and suggestions on topics you’re curious about or wish to delve deeper into. Together, we can demystify AI, making it accessible and engaging for everyone. Let’s continue this journey towards a better understanding of AI. Please share your thoughts with us via email: marty@bearnetai.com, and don’t forget to follow and share BearNetAI with others who might also benefit from it. Your support makes all the difference.
Thank you for being a part of this fascinating journey.
BearNetAI. From Bytes to Insights. AI Simplified.
Categories: Artificial Intelligence, Computer Science, Technology Ethics, Cognitive Science, Innovation and Research
The following sources are cited as references used in research for this BLOG post:
Artificial Intelligence: A Guide for Thinking Humans by Melanie Mitchell
Superintelligence: Paths, Dangers, Strategies by Nick Bostrom
Architects of Intelligence by Martin Ford
Human Compatible: Artificial Intelligence and the Problem of Control by Stuart Russell
The Master Algorithm: How the Quest for the Ultimate Learning Machine Will Remake Our World by Pedro Domingos
Machines of Loving Grace: The Quest for Common Ground Between Humans and Robots by John Markoff
Life 3.0: Being Human in the Age of Artificial Intelligence by Max Tegmark
© 2024 BearNetAI LLC